

However, as soil spectra are a comprehensive reflection of soil properties, the characteristic wavelengths of iron oxide are not the same in different regions and are easily masked by organic matter.
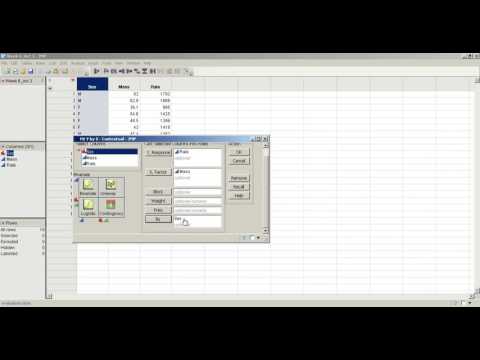
Of these pre-treatment methods, CR, log (1/R), SNV and CWT have all been widely used. Due to the presence of environmental and instrumental noise, spectral smoothing has become an essential step and other processing methods are based on spectral smoothing afterwards. Common spectral pre-processing methods include spectral smoothing (Savitzky–Golay filter, SG), continuum removal (CR), absorbance (log (1/R)), multiple scattering correction (MSC), standard normal variate (SNV), continuous wavelet transform (CWT) and differential transformations. Therefore, suitable spectral pre-processing is an indispensable step in soil hyperspectral modelling to improve the predictive power of the model. In practice, however, many factors can affect the quality of the spectra these include the complexity of the composition of the soil itself, the environment and the noise of the instrument itself. Hyperspectral, with its high spectral resolution and wavelength continuity, is widely used for the inversion of soil physicochemical properties. The traditional method for the determination of iron oxide content has a high accuracy but a high determination cost and a long cycle time. The results show that the FOD can significantly improve the correlation with iron oxide compared with the CR, SNV, log (1/R) and CWT the baseline drift and overlapping peaks decrease with increasing the order of FOD the CARS algorithm based on 50th averaging can select more stable characteristic wavelengths the FOD achieves better results regardless of the modelling method, and the model based on 0.5-order differential has the best prediction performance (R 2 = 0.851, RMSE = 5.497, RPIQ = 3.686). Finally, two regression models (backpropagation neural network, BPNN support vector regression (SVR) were used to predict the content of iron oxide. Secondly, competitive adaptive reweighted sampling (CARS) was used to extract characteristic wavelengths. In this paper, we first used conventional spectral transformation (continuum removal, CR standard normal variate, SNV absorbance, log (1/R)), continuous wavelet transform (CWT), and fractional order differential (FOD) transform to process original spectra (OS). However, due to the overlapping characteristic spectra of iron oxide and organic matter in the visible-near infrared, appropriate spectral transformation methods are important. Therefore, it is important to have information on the iron oxide content of soils. Iron oxide is the main form of iron present in soils, and its accumulation and migration activities reflect the leaching process and the degree of weathering development of the soil.
